Fragmented AI adoption
Unlike holistic artificial intelligence systems, many companies choose fragmented AI adoption. This approach involves implementing various independent AI solutions in specific areas of an organization, such as sales, marketing, production, or customer service, without attempting to integrate them into a cohesive system. While this strategy may seem quick and cost-effective, it often comes with significant limitations and negative consequences for the company.
Examples of Fragmented AI Adoption:
1. AI in Marketing:
- A company deploys an AI tool to automate advertising campaigns, personalizing content based on customer data. However, this data is not synchronized with other departments, such as sales or logistics. As a result, marketing may promote products with low inventory levels, leading to customer dissatisfaction and reputational damage.
2. AI in Customer Service:
- An AI chatbot is introduced in the customer service department but operates in isolation from CRM systems or sales databases. When a customer asks a question about a previous purchase, the chatbot lacks access to transaction history, resulting in low effectiveness and user frustration.
3. AI in Logistics:
- The logistics department uses an AI tool to optimize delivery routes, but the solution does not consider dynamic demand changes managed by the sales department. As a result, optimized routes fail to meet actual market needs, causing inefficiencies or delays.
4. AI in Human Resources:
- An AI system is implemented to analyze resumes during recruitment but functions independently of the broader HR infrastructure. This recruitment system does not consider actual needs arising from the company’s development plans, potentially leading to hiring individuals misaligned with long-term organizational goals.
Problems Resulting from Fragmented AI Adoption:
1. Lack of Data Consistency: Each AI tool operates on separate datasets, making it impossible to achieve a comprehensive view of the company’s operations. Data remains scattered, leaving much of its potential untapped.
2. Process Isolation: Fragmented AI tools work independently, leading to uncoordinated activities across different departments. This often results in conflicts of interest and resource wastage.
3. Increased Maintenance Costs: Implementing multiple AI tools requires separate management, technical support, and integration efforts, driving up costs and operational complexity.
4. Scalability Challenges: Fragmented solutions are often not designed for collaboration with new technologies. As the company grows, the lack of integration becomes an increasingly significant constraint.
5. Inefficient Decision-Making: With data and AI operations fragmented, organizations lose the ability to make decisions based on a complete picture of their situation. This can lead to short-sighted decisions and financial losses.
The Alternative: A Holistic Approach to Counter Fragmentation
Instead of adopting AI tools in a fragmented manner, companies should aim to create integrated, holistic AI systems. This approach allows for the full potential of artificial intelligence to be realized, minimizes problems caused by isolated data and processes, and supports a long-term strategy for organizational development.
Holistic AI systems integrate all tools and data into a unified whole, enabling better coordination between departments and faster responses to market changes. As a result, organizations become more efficient, flexible, and competitive.
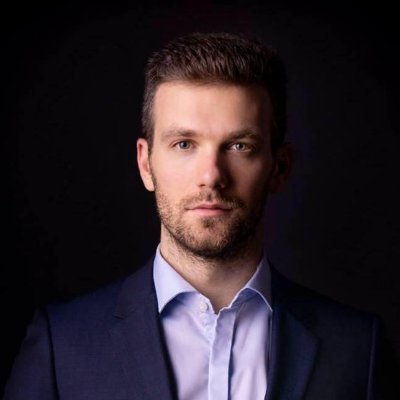
Michal Szymanski
Co Founder at MDBootstrap , CogniVis AI and AIFor.Biz / Listed in Forbes „30 under 30" / EOer / Open-source and AI enthusiast / Dancer, nerd & bookworm.
Author of hundreds of articles on AI, programming, UI/UX design, business, marketing and productivity. In the past, an educator working with troubled youth in orphanages and correctional facilities.